Neural Cameras
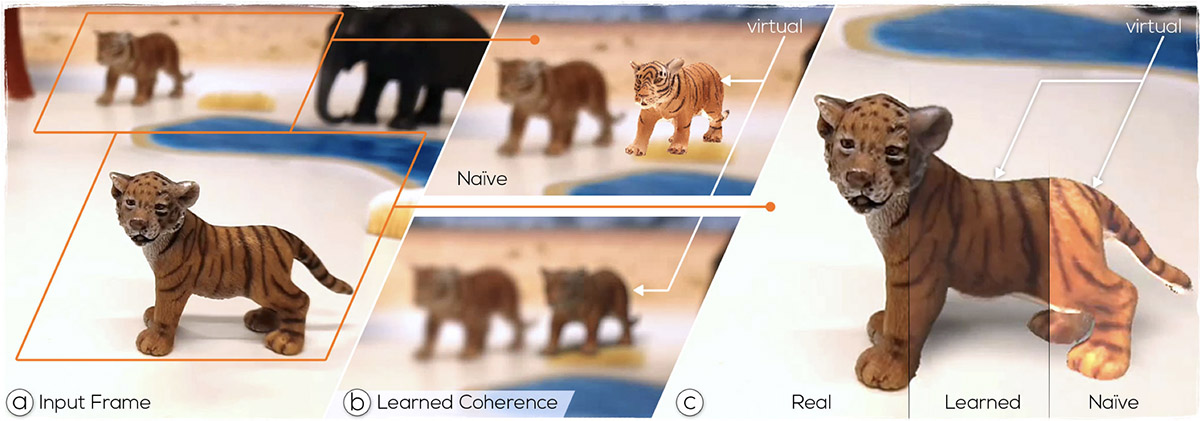
Overview: Learned visual coherence for simulating the characteristics of physical cameras. (a) Input frame showing a scene capture with a physical camera. (b, top) Inserting a virtual object (the tiger on the right side) using a na ̈ıve rendering introduces a break-in visual coherence. (b, bottom) We address this gap with a Neural Camera which is able to reproduce characteristics such as blur and color filtering. (c) A direct comparison between a na ̈ıve rendering of a virtual object (right-Naive), our approach (middle-Learned), and to the output of a real camera (left-Real).
Abstract: Coherent rendering is important for generating plausible Mixed Reality presentations of virtual objects within a user’s real-world environment. Besides photo-realistic rendering and correct lighting, visual coherence requires simulating the imaging system that is used to capture the real environment. While existing approaches either focus on a specific camera or a specific component of the imaging system, we introduce Neural Cameras, the first approach that jointly simulates all major components of an arbitrary modern camera using neural networks. Our system allows for adding new cameras to the framework by learning the visual properties from a database of images that has been captured using the physical camera. We present qualitative and quantitative results and discuss future direction for research that emerge from using Neural Cameras.
Acknowledgements: This work was enabled by the Austrian Science Fund FWF (grant no. P30694), MBIE Endeavour Smart Ideas, the German BMBF in the framework of the international future AI lab ”AI4EO” (grant no. 01DD20001), and the Competence Center VRVis. VRVis is funded by BMK, BMDW, Styria, SFG, Tyrol and Vienna Business Agency in the scope of COMET - Competence Centers for Excellent Technologies (879730) which is managed by FFG.
Video: